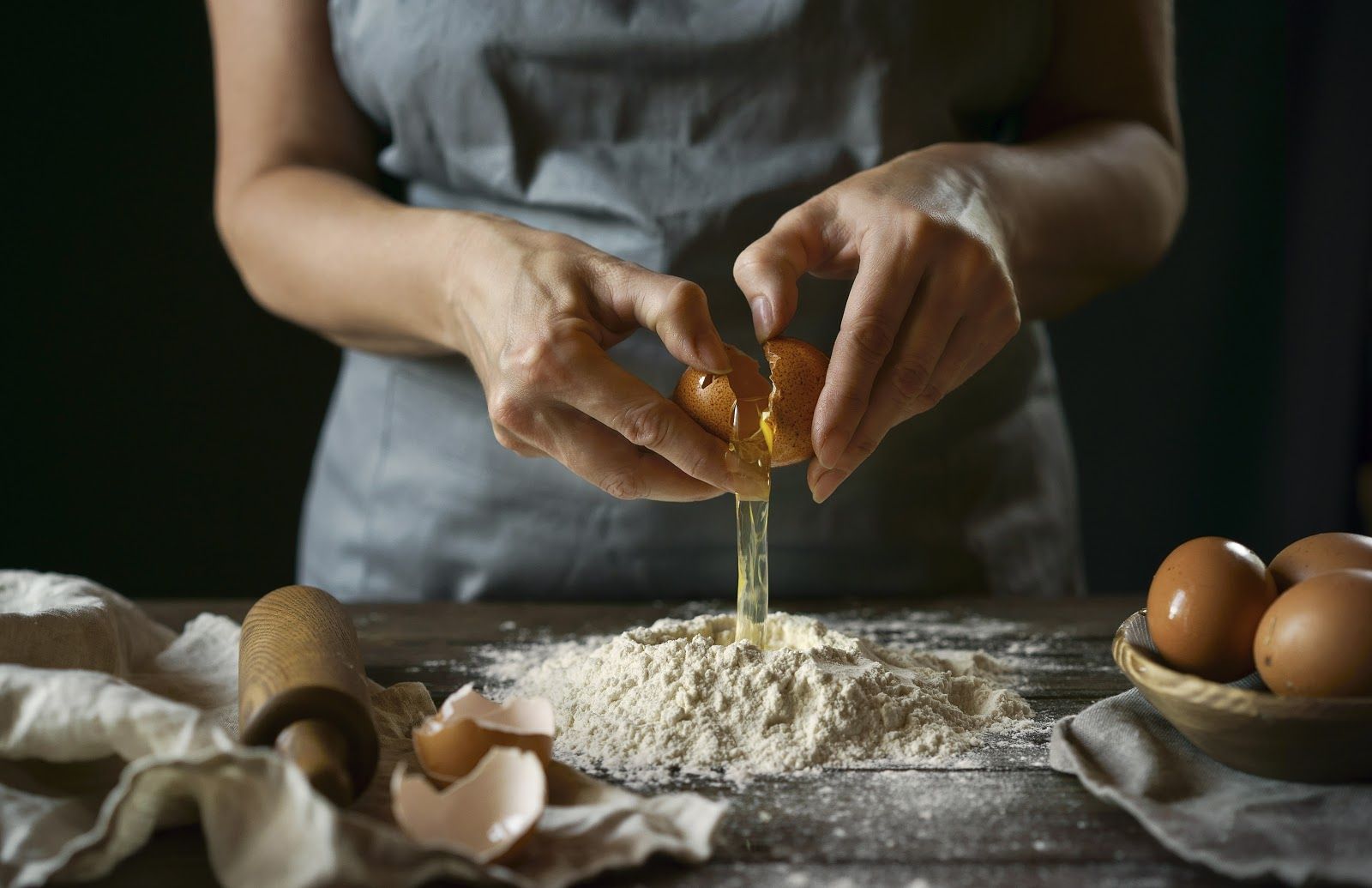
Recently, Gartner published their annual Magic Quadrant for Data Science and Machine Learning Platforms (DSML) 2019. Many businesses use this report to help shortlist vendors for consideration when beginning new projects or making purchase decisions. This year’s analysis has seen seismic shifts in the positions of many vendors and Gartner states that this is a “dynamic and rapidly evolving market.”
We are pleased to announce that we are a Leader in the Gartner Magic Quadrant for Data Science and Machine Learning 2019.
When evaluating DSML platforms, organizations must not only consider the data acquisition, data prep, and model-building process but also realize the importance of the model operationalization process or the act of rolling out data science into your entire organization. Organizations must have a strategy for each of the following for their data science initiatives to be successful:
- Business Process
- Machine Learning Process
- Model Operations and Governance Process
I refer to this as ML Ops. Gartner agrees about the importance of ML Ops by stating that “The full benefit — including business value — of data science and ML will not be achieved unless models are both: 1) Embedded in business processes, and 2) Maintained, monitored, and managed over time.”
All too frequently, when I speak with clients and prospects about their initiatives, they almost always eagerly talk about the projects underway that utilize various open source languages like R, Python, and SQL; libraries like scikit-learn, Spark MLlib, and H20; and frameworks like TensorFlow, SageMaker, and AzureML. They are focused on the math, and when you ask about their plan for operationalizing (deploying, monitoring, refreshing, and maintaining their machine learning pipelines), they usually haven’t fully thought through that process.
Gartner goes on to say that over 60 percent of models are never operationalized. Wow, 60 percent! That means over half of the companies that are deploying machine learning models are not operationalizing them or able to apply them to the business. No wonder that businesses can be skeptical about the value of data science and machine learning.
Operationalizing analytics helps companies realize the benefits of data science
To help orchestrate all of the various open-source technologies and components that are needed to realize the value of data science, many clients use Spotfire® Data Science to help govern, deploy, and manage data science pipelines within their organization.
We understand the value that open source brings to organizations but also the complexity that might be involved. Open source provides flexibility, extensibility, choice, and of course, access to the latest innovations. But if you don’t have the right operations in place to make it work correctly, you won’t get the full benefit of data science.
I like to use the analogy of making bread in your home. It’s fairly simple and requires only four ingredients: flour, water, yeast, and a bit of salt. You put everything in the bowl, mix it up, let it rise, then cook it in the oven. A word of caution though, if you don’t follow the recipe to a T, you may end up with inedible bread. This is the same with machine learning, and many organizations need help getting it just right.
Further, if a company were to treat analytics as a core business process, as they should, they must quickly turn their home kitchens into bakeries. To do this successfully, they’d probably need industrial mixers, larger quantities of ingredients, more ovens, a distribution network, quality control processes, more bakers, and so on.
The point is: when you want to scale analytics across an organization, you need to focus on the Business Process, the Machine Learning Process, and the Model Operations and Governance Process (aka ML Ops).
Organizations that are successful with data science have been able to orchestrate the people, process, and analytic technology for competitive advantage. Customers like Tipping Point who are helping to reduce socioeconomic inequality, customers like the Univ. of Iowa Hospitals and Clinics who are reducing surgical site infections, and Leidos who are doing work with the CDC and NASA.
Contact us to see how we can help you get your Spotfire Data Science initiatives off the ground.
Gartner does not endorse any vendor, product or service depicted in its research publications, and does not advise technology users to select only those vendors with the highest ratings or other designation. Gartner research publications consist of the opinions of Gartner’s research organization and should not be construed as statements of fact. Gartner disclaims all warranties, express or implied, with respect to this research, including any warranties of merchantability or fitness for a particular purpose.