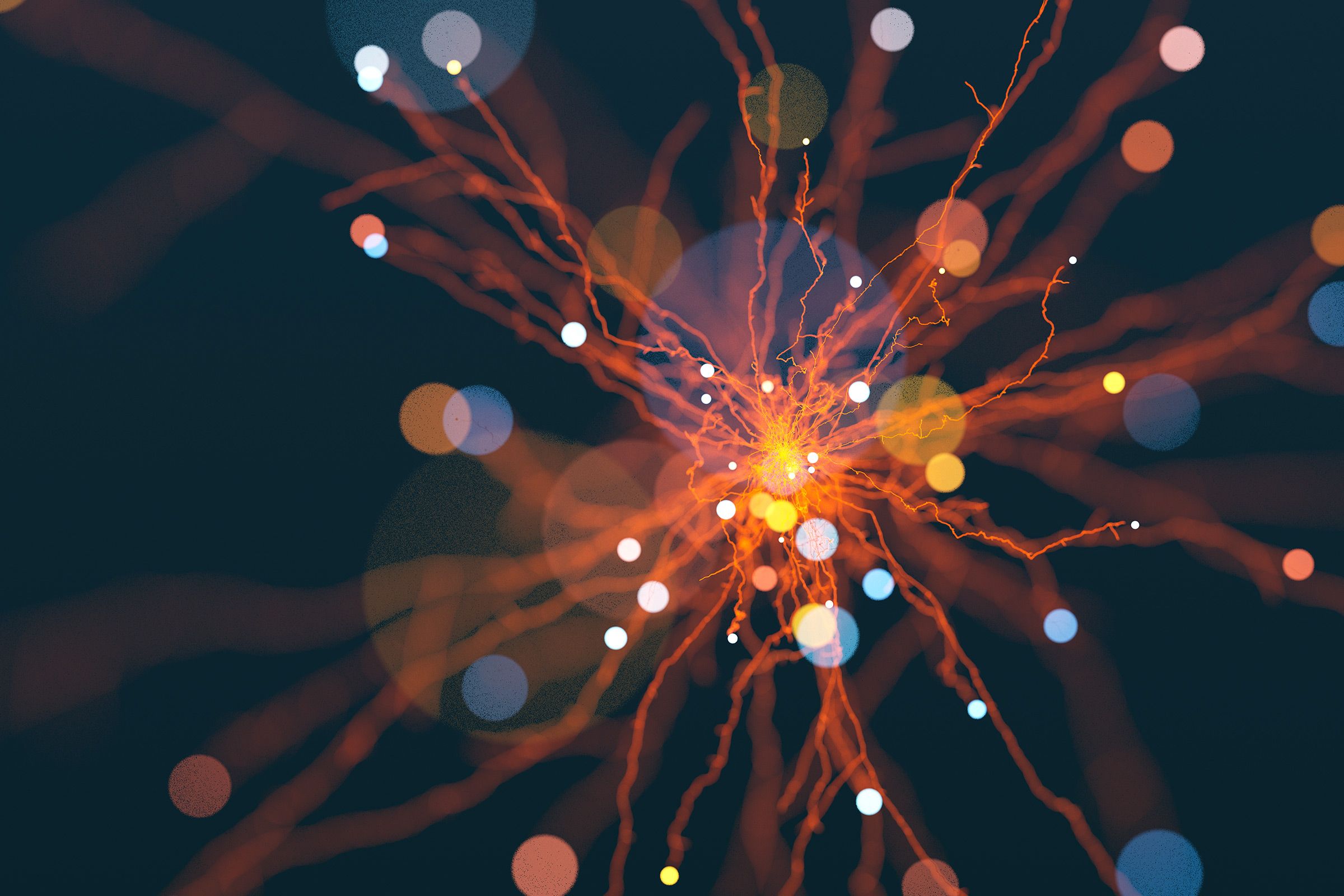
Today there are 275 unicorns, private companies with over $1 billion in valuation, around the world. These companies are changing the way we do business, requiring everyone to adopt a more accelerated pace of innovation to create hyper-personalized customer experiences—all built upon a more effective use of data and technology.
To keep up and maintain a competitive advantage, you need to ensure your technology investments are delivering the most value possible for your company.
While many organizations are investing heavily in data science and machine learning (ML), far fewer have found a way to monetize their initiatives and fully realize the value from the insights uncovered.
But, why aren’t organizations able to monetize their ML initiatives?
The reason is simple: organizations today often fail to operationalize machine learning models by integrating them into their business processes.
If you’re struggling to accomplish this, you’re not alone. According to the Gartner Data Science Team survey, conducted at the end of 2017, even within organizations benefiting from the expertise of mature data science teams, less than half of data science projects end up being fully deployed. Another survey conducted in 2017 points to the fact that this phenomenon is not unique to data science, and that deploying AI projects into business processes or applications remains the principal barrier to delivering business value. See Gartner’s survey results below.
Best practices to operationalize data science and machine learning
To realize the value from your machine learning initiatives, you need to focus on ML Operations to manage the end-to-end ML production lifecycle. Here’s some best practices to keep in mind:
- Focus on the decision and communicate: Setting up your machine learning initiatives for success begins before the real work even starts. Focusing on what business decision you’re trying to make and working backwards from that is the first step. If you could predict something 1 minute, 1 week, or 1 month into the future, how would your business process change? Once you’ve done that, assemble a cross-functional team consisting of business experts, data science, data engineers, and IT and communicate to make sure everyone is in alignment and working toward the same goal.
- Establish a CoE and set guidelines: A common struggle for organizations trying to operationalize their machine learning models is a lack of internal structure. When there’s no set structure or best practices to follow, different teams within the business will keep reinventing the wheel, trying to solve the same problem in multiple ways. That’s why many organizations establish a Center of Excellence (CoE) to communicate best practices, create training programs, and share reusable templates and guidelines across the organization. Having structure actually opens up room for collaboration by eliminating confusion and allowing creative people to eliminate the more mundane tasks and focus on being innovative and solving new problems.
- Optimize people, processes, and technology: Data science is a team sport. To ensure success, you must facilitate handoffs within the team and between teams. Furthermore, “DIY data science is not scalable”. Just like you may be able to make a loaf of bread at home in your kitchen but need more advanced equipment to run a bakery, open-source data science doesn’t offer all the functionality needed to manage the complexity of machine learning projects.
Monetize and realize the full value of Spotfire® Data Science and machine learning initiatives that can operationalize ML at your company.