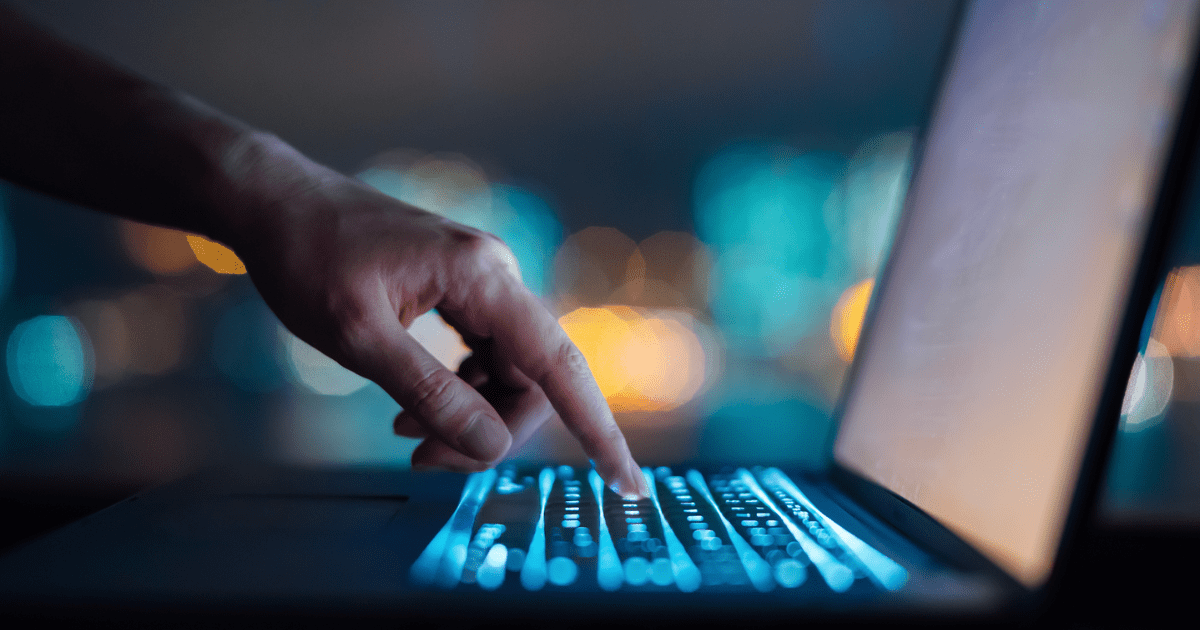
Enterprises around the globe continue to focus on digital transformation, and generative AI (Generative Artificial Intelligence) has a potential role to play in accelerating the organization toward its goals. In this blog, you’ll learn more about generative AI, three high-value use cases for this emerging technology, and what risks to manage as you begin exploring generative AI for business use.
Generative AI Compared to Conventional AI
Artificial intelligence (AI) is, broadly speaking, the research towards and development of computer systems to automatically perform tasks normally performed by human capabilities, including speech recognition, visual perception, language translation, and decision-making. Generative AI is a subset of AI that relies on machine learning, a data science discipline that develops AI models which use data patterns to “learn” without human direction. The ongoing generation of vast amounts of data in enormous volumes and varieties across enterprises is fueling advances in machine learning. One of these advances is generative AI, an algorithm-based system that takes autonomous action based on training data.
Unlike conventional AI, which has often been used to identify or categorize data, generative AI produces outputs—images, text, computer code, and even audio—based on its training datasets. Generative AI systems, broadly speaking, complete outputs based on what are “most-likely-comes-next” patterns in response to prompts. For example, conventional AI could be trained to identify artifacts in mammogram images that indicate hard-to-detect cancers. Generative AI could be trained and then prompted to synthesize (generate) new images with similar artifacts as well as “false positive” artifacts to help train medical students on what to look for when they review mammogram images. It is beyond the scope of this blog to dive into the technology behind generative AI, such as Generative Adversarial Networks (GANs) and Variational Autoencoders (VAEs), but you can learn more here.
Generative AI has captured the imagination of the public as well as the executive suite. IT and data leadership should expect business leaders to bring generative AI up for discussion—and value exploration—if those conversations haven’t happened yet.
3 Digital Transformation Use Cases for Generative AI
The current hype around generative AI can obscure the real business value worth exploring now. Unlike the current moment’s focus on the use of generative AI for personal content creation, real business value can be found in several use cases:
Customer Experience (CX)
McKinsey reports that enterprises using digital transformation applied to CX lead in value creation and increased revenue from existing customers, generating “nearly double the shareholder value than their industry peers, on average.” Future-forward businesses can now explore another way to leap ahead through generative AI. They can transform manually-maintained, article-based, hierarchical Help, and FAQ experiences into easier-to-update and easier-to-consume personalized chat-based help. By applying open-source generative AI technologies—for example, deploying open-source LLMs to private endpoints that can access and aggregate a company’s own knowledge article repository—the customer no longer needs to crawl drop-down menus or search multiple articles to find the information they need. They can use a single chat interface to answer the question “What do you want to do or learn today?” and see aggregated information from across the organization in a friendly, conversational format.
Manufacturing and Supply Chain Resilience
Additive manufacturing, or custom-printing of 3D parts, is a strategy increasingly used to improve supply chain resilience. Mark Cotteleer, Managing Director of Supply Chain and Operations Consulting at Deloitte, stated in TCT Magazine that “‘additive manufacturing is…the quintessential example of digitally distributed manufacturing…it brings together all the interesting attributes of digital distributed manufacturing [including] the ability to work across the supply base and distribute production to the point of demand at the time of demand… .’”
Generative AI can help turn that approach to resilience into innovation—by using generative design AI to autonomously prototype optimized 3D-printed parts, time-to-optimization can be radically decreased, and the optimization benefits can be significant. As Ryan McClelland, a research engineer at NASA’s Goddard Space Flight Center, noted in a recent Fast Company article, “Using generative AI to design the parts shaved off a third of their typical weight without sacrificing performance.” If your enterprise needs more supply chain adaptability, generative AI is well worth exploring.
Operations Optimization
Whether DevOps, FinOps, or any Ops, operations tasks with multiple manual steps may benefit from the use of generative AI. For example, DevOps best practices require good technical documentation to be created for new applications and capabilities. Through iterative prompts, generative AI tools can rapidly turn silos of notes and code into review-ready draft documentation, saving hours per document, as discussed here. Similarly, FinOps requires the aggregation of data from disparate cloud billing systems into a single source of truth, and the use of open-source LLMs with private endpoints can accelerate that process by automating resource tagging, freeing up valuable person-hours.
These three use cases are not industry-specific, but there are numerous applications where generative AI might similarly automate and accelerate first draft or prototype creation across a wide variety of industries. However, like any new and disruptive technology, the use of generative AI is not risk-free.
Generative AI Risks
The risks associated with generative AI are similar in many respects to those of conventional AI:
- The need for well-governed, quality data. Especially for those enterprises that will explore open-source generative AI tools, and thus need to supply their own training data, you must ensure that data is clean, reliable, and relevant to the use case.
- The risk of bias. Generative AI requires vast volumes of training data, and there has been much written about the inadvertent incorporation of bias in those training data sets, whether from historical structural bias, inadequate planning for community input, or the siloing of data scientists. The outcomes from baked-in bias can create an existential threat to your organization’s reputation, but you can manage that risk with best practices.
- The need for internal expertise. Even if your company outsources generative AI projects, it is still legally responsible for the systems and their outputs. Like best practices for conventional AI use, generative AI outputs always require review by subject matter experts. According to one leading vendor of generative AI systems, “Great care should be taken when using language model outputs, particularly in high-stakes contexts, with the exact protocol (such as human review, grounding with additional context, or avoiding high-stakes uses altogether) matching the needs of a specific use-case.”
There are at least three areas where the use of generative AI poses new risks that must be addressed:
- Copyright. There are active lawsuits in progress over whether there has been improper use of copyrighted material in training data. Before embarking on a pilot program for generative AI, seek guidance from your legal team. It may be best to develop a pilot using open-source generative AI tools with your own datasets to reduce risk.
- Intellectual property. Generative AI is upending the ability of enterprises to protect their IP. For example, a popular use case for generative AI has been for code optimization and debugging. However, the information entered into the chat box for popular generative AI tools may be consumed by the AI tool company for use in training data, posing risks to IP ownership and confidentiality. Further, bad actors could use generative AI to mass-generate “prior art” and block competitors from obtaining patents.
- Regulatory compliance. For global enterprises, generative AI use may be prohibited or subject to regulation in certain countries. Recently, the Italian Data Protection Authority (IDPA) ordered OpenAI, the maker of ChatGPT, to stop processing user data as it investigates possible GDPR violations. Privacy regulators in Germany, France, and Ireland may follow the IDPA in blocking ChatGPT over privacy and security concerns.
As with any new, disruptive technology, the benefits of generative AI may strongly outweigh the risks—but only your organization, and its trusted legal advisors, can determine what’s needed to move quickly and safely towards those benefits.