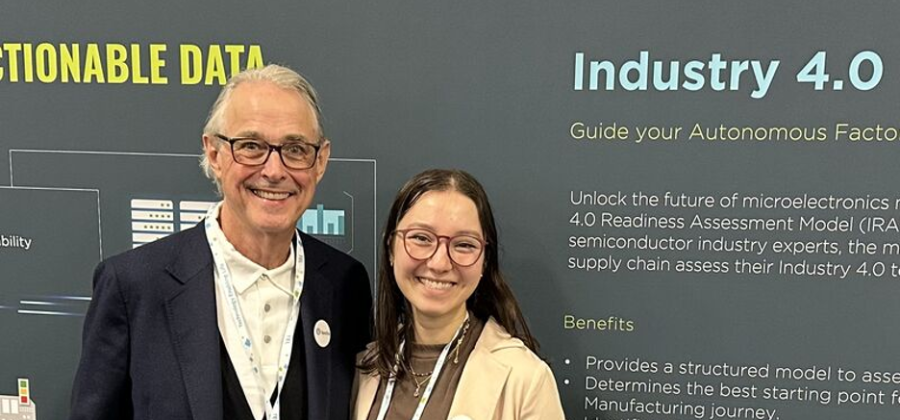
SEMICON West, which was held this summer in San Francisco, California, provided a large opportunity for the semiconductor and microelectronics manufacturing industry to come together and discuss the challenges and future opportunities within the industry. The overarching theme of this year’s conference was the large amount of growth the industry was seeing. This was reflected in the increase in attendance, up 35 percent from last year, as well as the growing number of funding opportunities, made largely available through the CHIPS and FABS act. Several major companies, such as Samsung, TSMC, and Intel, are expanding and building new fabs in the US as a result of these funding opportunities.
The CEO of SEMI also reported that artificial intelligence is contributing largely to the growth of the industry; it is predicted that by 2030, semiconductor manufacturing will be a $1 trillion industry. Due to this trend, there was also a large emphasis on the importance of having and executing strong digital transformation initiatives within high-tech manufacturing companies. At SEMICON West, several sessions and exhibits focused on the integration of machine learning and artificial intelligence into various manufacturing processes.
Yield optimization using AI
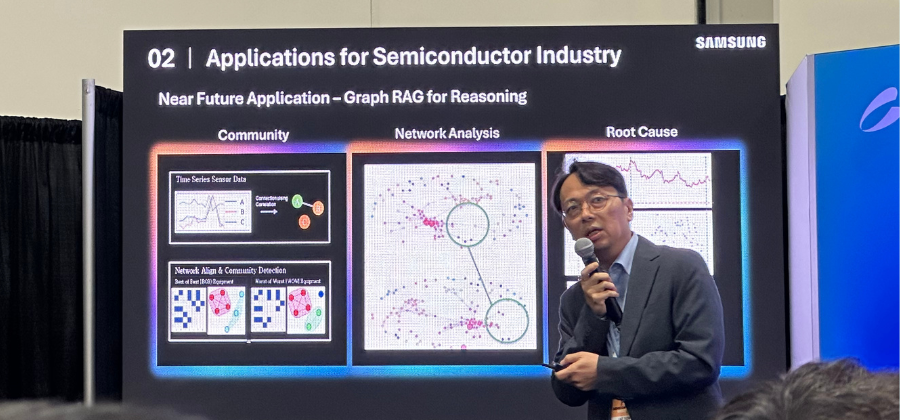
Presentation of Jae-Yong Park, VP of Technology at Samsung Semiconductor
Front-end semiconductor manufacturing refers to the wafer fabrication process, with the largest success metric being yield (i.e. the percentage of accepted die in a wafer). Many techniques and technological advancements for optimizing yield using AI were discussed at SEMICON West.
Throughout the front-end manufacturing process, multiple types of data are often generated, ranging from tabular, to text, to image data, and more. This was one of the primary challenges discussed—how can organizations effectively utilize a variety of data types? Regardless of the type of data, data is largely underutilized in the industry; roughly 80 percent of data generated never even gets looked at, as noted by several presenters. One way that data can be more effectively utilized is through the use of multimodal foundational models. These models can handle different data types, and be fine-tuned for specific use cases, such as performing root cause analysis, process optimization, and defect detection and classification.
One use case that was presented by multiple speakers, like Siemens and Gauss Labs, was utilizing multiple data types and digital twin technology to perform virtual metrology. Virtual metrology harnesses sensor measurement data to predict various properties of wafer, and therefore predict product quality. Traditionally, physical metrology would only utilize measurements from 1-5 percent of wafers, while virtual metrology can be used for 100 percent of wafers, resulting in faster and more accurate quality predictions, according to Gauss Labs.
The announcement of SemiKong, the first open-source LLM for semiconductor manufacturing, affirmed the growth of AI, and more specifically generative AI, in the industry. Announced by Aitomatic, SemiKong was trained on industry-relevant materials and was built on top of domain-specific agents, which allow planning and reasoning to occur outside of the LLM. With this approach, SemiKong was shown to outperform vanilla LLMs when it came to answering technical questions regarding semiconductor manufacturing.
AI for back-end semiconductor manufacturing
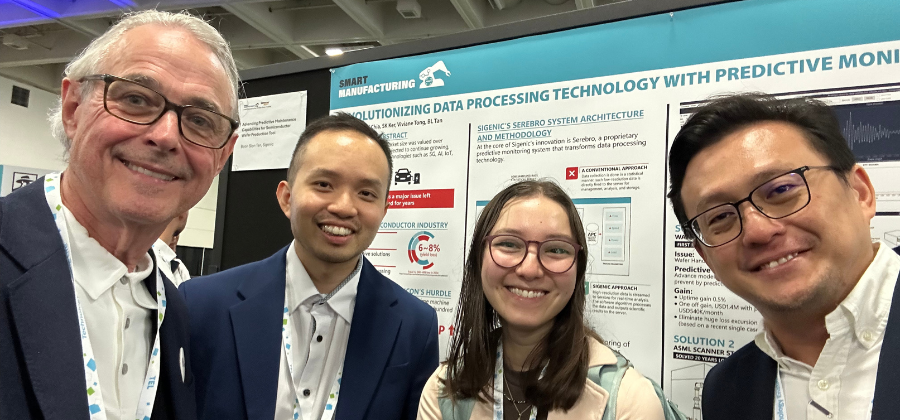
Spotfire’s Michael O’Connell and Evie Klaassen with SK Ker and Boon Siong Tan from Sigenic Pte Ltd.
Back-end semiconductor manufacturing refers to the assembly, packaging, and testing of an integrated circuit, and occurs after the wafer fabrication process. The Smart Manufacturing Pavilion at SEMICON West discussed a number of topics covering this part of the manufacturing process, and how AI can provide additional improvements and opportunities to achieve zero defect manufacturing.
OEE, or Overall Equipment Effectiveness, is a long-standing metric used to measure manufacturing productivity. However, as discussed by folks from IBM Bromont, Kulicke & Soffa, and Tignis, measuring OEE often requires a large amount of manual input, which can result in slower implementations of process improvements and increases in cost. With AI, not only can performance data be measured automatically, allowing for OEE metrics to be analyzed closer to real-time, but other maintenance steps can be automated based on OEE, such as ordering new parts or alerting technicians of unexpected failures as soon as they occur.
Anomaly detection also plays a critical role in reducing manufacturing defects and performing failure root cause analysis. ASMPT presented on how AI can aid in anomaly detection. By utilizing robust models and real-time data, defects can be detected earlier, and therefore manufacturing issues can be solved faster, further preventing future defects from occurring.
Gain industry-specific insights
Is your company ready to utilize OEE metrics or have access to anomaly detection analysis? With Spotfire, you can! To learn more about Spotfire® for Manufacturing, check out our high-tech manufacturing solutions. To download our Manufacturing extensions for Spotfire, visit the Spotfire Community.