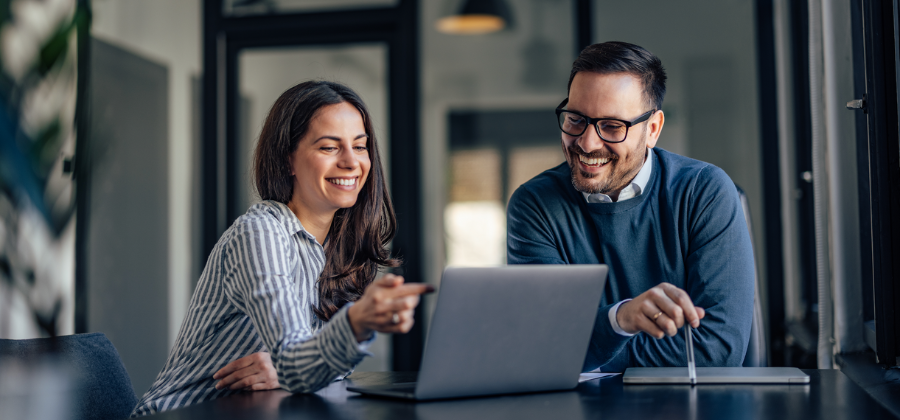
A long-ignored technology gap exists between analytics and business intelligence (ABI) platforms and data science and machine learning platforms (DSML). Leaving many subject matter experts, data scientists, and business users disconnected from highly valuable predictive outcomes and advanced analytic insights.
Many ABI solutions have focused on an 80/20 approach to the market, lowering the cost of entry but only solving 80 percent of the challenges. At the same time, ignoring the 20 percent that is often highly valuable, complex, and industry specific. In some instances, DSML functionality has moved closer to traditional ABI users, but the sophisticated statistical and algorithm functions are owned by data scientists, causing a dependency that slows the time to insights.
From the data perspective, many traditional ABI platforms rely on stand-alone data management solutions and more often exist separately from DSML platforms and the experts that operate them. Collaboration is also difficult and time consuming across these silos, slowing time to insight and action for users.
So, how do you combine the deep insights and actions of data science with the adoptability and engagement of an ABI platform—plus scale it across your organization? The answer is, visual data science platforms.
Bridging the gap
Visual data science platforms deliver a set of differentiating features that address these gaps, making these solutions uniquely valuable as part of a company’s data and analytic strategy.
Here are a few of the competencies found in visual data science platforms:
- A visual-first approach to advanced analytics
- Industry-specific, domain-expert focused
- A seamless interaction with data
- One platform for all
Let’s dive into each of these areas to explore why these solutions can augment and improve your approach to data and analytics.
Visual-first approach
Data science has always been statistics and algorithms first and often developed in a silo. Visual data science platforms begin with highly interactive visualizations that leverage statistics and algorithms, reducing the gap between business users and data scientists. There is exceptional value in delivering data science-driven insights without the PhD level challenges and hurdles. Enabling users to leverage insights that incorporate data science outputs like cluster and regression analysis or understanding complex data patterns and relationships via visual exploration speeds time to insights and delivers value. Integrated within this visualization-first approach, users can directly explore the visual representation of the data and workflows as well.
Industry-specific, domain-expert focused
Domain experts are often challenged to find complex industry-specific visualizations that speak directly to their industry. For instance, visualizations for microprocessor manufacturing, machine maintenance processes, or oil well performance visualizations that are coupled to geological data. Visual data science platforms focus on these types of use cases, serving the specific needs of domain experts while making it easier to leverage sophisticated data science functions. Visual data science platforms engage a new group of business and technical users with deep industry-specific domain expertise that benefit from the visual UX and the speed it delivers.
Seamless interaction with data
Data is the foundation of advanced analytics and business insights. Interacting with visual representations of data enables business users and SMEs to move at the speed of thought regarding data. Orchestrating, cleaning, preparing, and transforming data should be an immersive experience. Visual data science’s highly interactive visualizations can quickly enable experts to identify data inconsistencies, data quality issues, and incorporate additional data on the fly to enhance and add value to projects within a single platform environment.
One platform for all
Working within a single environment to leverage advanced analytics, understand, and work with data while collaborating with team members is a critical function of visual data science platforms. The single integrated platform saves time, eliminates complexity, and enables the natural flow of analytic discovery and application design. It’s important that these unified platforms are also extensible—and integrate with other applications and new data sources. It’s also critical that this openness and extensibility includes a logical path for data scientists to include programming languages like R and Python and user interfaces such as Notebooks into the platform. Bringing business, subject matter experts, and data science teams together on one platform to drive highly valuable insights and actions delivers significant value for enterprise companies.
I’ll be sharing my perspective on visual data science platforms in upcoming posts. I look forward to your feedback and questions.
Shawn Rogers, CEO BARC US – Industry Analyst