What is data wrangling?
Data wrangling is the process of bringing together data from a variety of data sources and cleaning it for easy access and analysis. The amount of data being collected today is growing rapidly, requiring organizations to implement processes for handling and organizing it with the ultimate goal of simplifying data preparation workflows.
Successful data analytics depends on organized, accurate, and actionable data. But studies show that 50–80% of analysis time is spent wrangling data that has errors, inconsistencies, and poor organization for analysis. The top data wrangling solutions today allow for automated, inline data wrangling that lets you connect, blend, clean, and wrangle data from any source including big data sources.
The first step in analytics is gathering data. Then as you begin to analyze and dig deep for answers, it often becomes necessary to connect to and mashup information from a variety of data sources. Data can be messy, disorganized, and contain errors. As soon as you start working with it, you will see the need for enriching or expanding it, adding groupings and calculations. Sometimes it is difficult to understand what changes have already been implemented.
Moving between data wrangling and analytics tools slows the analytics process—and can introduce errors. It’s important to find a data wrangling function that lets you easily make adjustments to data without leaving your analysis.
The benefits of data wrangling
Access and link any data source
Today’s top data wrangling solutions allow you to connect all of your data from a variety of sources. By mashing up and matching your data, whether structured or unstructured, you can gain a clearer, more complete view of the data and generate insights.
Spend more time analyzing data
Instead of spending countless hours trying to organize your data before you can even begin to make sense of what it means to your business, use a data wrangling solution to save time and money. Then you will be able to focus on deeper analysis, spend more time on data exploration, and spark insights that can be used for business improvements.
Ensure trustworthy data
Data wrangling adds credibility to your data. By cleaning and organizing all of your data, you can be sure that the analysis that follows yields accurate results that can be acted upon without question.
Easy access and collaboration
By simplifying your data, data wrangling allows for easier access to a wider audience within your organization. Making your data easier to understand opens the discussion to non-experts, enabling faster decisions and richer collaboration between teams.
Essential data wrangling capabilities
Fast and easy inline data wrangling
Today’s top data wrangling solutions allow you to perform data preparation and analysis in the same platform, and in the data source itself. Inline data wrangling lets business users make adjustments: Mashup columns and rows from various data sources; unpivot with one click; change the data type, category, and column name; dynamically group columns from visualizations; modify sort order; split smart columns; and cleanse data by replacing wrong or missing values. Full API support lets you insert functions, like adding or changing join types to bring deeper insights.
Auto-recording each of your steps
The best data wrangling solutions automatically build a data pipeline on the source view data canvas that documents all the steps you take in data wrangling and analysis. This way traceability and auditability of the data model can be ensured and easily shared, with information about the data sources, connections, operations, and data transformations automatically recorded.
The impact of data wrangling
Data wrangling is an essential step in ensuring that you get valuable, accurate insights from your data during analysis. Data wrangling helps transform your messy, complex, or incomplete data into actionable information that is easy to use. With the mountains of data that organizations are dealing with today, data wrangling is necessary to separate relevant data from the rest. Data wrangling protects companies against untrustworthy data, helping to make sense of complicated datasets and determine any inconsistencies or errors that need to be changed.
Efficient data wrangling can help analysts spend more time on actually analyzing data. Instead of spending the majority of the time trying to organize and clean data before starting analysis or drawing any insights, analysts can focus on driving better decision-making based on accurate data.
Data wrangling can also help open collaboration to more employees, even those who are not data experts. By simplifying complex datasets, data wrangling can make it easier to understand the meaning behind the data. With more collaboration on the data, organizations can deliver valuable insights to a wider audience and take action faster.
Getting value from data wrangling
Top data wrangling solutions today allow you to fix your data interactively while you analyze it, eliminating the back and forth between data preparation and analysis. This integrated approach to data preparation and analytics is easier to use, allows for rapid data cleansing, and is cost-effective.
Data preparation is always needed before analysis, but you almost never know what to do before examining the data. As you make changes, it’s important to validate them. Data wrangling can offer a visual overview of data sources, connections, operations, and transformations in a schema diagram. Whether you’re cleaning and combining data from multiple sources, or enriching and transforming it, you can view detailed information about the data operations that have been made and can preview the results. This enables you to establish and manage best practices for data wrangling and stay agile while maintaining governance.
What are some top use cases?
- Marketing Analytics: Modern marketing relies on data to accurately target potential customers, personalize customer experiences, and drive customer loyalty. But as IoT devices grow more popular and organizations begin tracking larger and more complex amounts of data on their customers, marketers need data wrangling tools to handle all that data. Once data wrangling is completed, marketing departments can analyze the data and make smarter, data-driven decisions.
- Machine Learning Applications: While machine learning and artificial intelligence (AI) continue to grow in popularity, organizations still struggle to ensure high data quality for accurate models. To combat this issue, businesses need to employ data wrangling solutions to bring together data from multiple, disparate sources and allow for scalability for big data.
- Healthcare Systems: The healthcare industry has become increasingly data driven, implementing analytics to drive efficiency and ensure the highest quality of patient care. But to achieve these results, healthcare providers must wrangle large amounts of data from medical records, patient data, demographic information, and research findings.
- Financial Services and Banking: Financial services and banking today rely on data to drive customer relationships, improve operations, and provide excellent customer service. To do this, financial institutions must wrangle transactional and customer data to maintain a competitive advantage. This is also important for detecting fraud or risk and meeting compliance requirements.
- Travel and Hospitality: Big data in the travel and hospitality industry opens up new opportunities for companies that can leverage it. Businesses that use data wrangling to collect and analyze customer data will be able to create engaging customer experiences and improve operational efficiency.
- Voter and Election statistics: Today’s elections rely on data to engage with voters, understand key issues, and develop a campaign strategy. To create a data-driven political campaign strategy, however, there is a lot of data wrangling involved to ensure accurate predictions.
Common data wrangling sources
- Unstructured data
- Structured data
- Quantitative data
- Qualitative data
- Big data
- Machine data
- Real-time data
- Open data
- Operational data
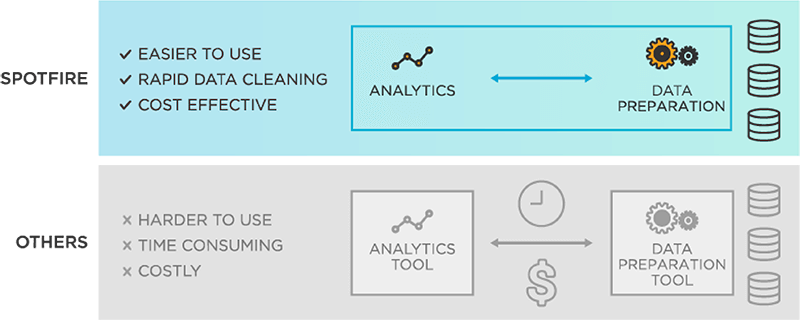