What is manufacturing analytics?
Manufacturing analytics is the use of operations and events data and technologies in the manufacturing industry to ensure quality, increase performance and yield, reduce costs, and optimize supply chains. Manufacturing analytics are part of a wider revolution known as Industry 4.0 where factories are expected to evolve into self-running and healing entities by embracing new technologies such as cloud and the Internet of Things (IoT).
Historically, manufacturers could not harness and use all of the data that was coming from the end-to-end manufacturing process, from supply chain to production to delivery to customer usage. They would have to rely on very complex, expensive tools that could only collect information from operators or machines. It could take weeks to identify why a manufacturing process was breaking down. In today’s highly competitive world, waiting weeks or even days for an answer is no longer sufficient. And, manufacturers need complete visibility into the entire process, from supply to the end customer, in order to get a 360 degree view and truly optimize their outputs.
Manufacturing analytics relies upon predictive analytics, big data analytics, the industrial internet of things (IIoT), machine learning, and edge computing to enable smarter, scalable factory solutions. With manufacturing analytics, you get actionable insights in real time. And, you only have to purchase one single software package to address all of your needs. Manufacturing analytics are purpose built to collect and analyze the data from an unlimited number of sources to identify areas for improvement. They span from machines to people, from an incoming order to the delivery of that order. Data is gathered and reformatted in an easy to understand way to show where there are issues along the process. Manufacturing analytics collects and manipulates large amounts of data to show insights that you can then act on or set up automatic business processes to respond to in real time.
Benefits of manufacturing analytics
Manufacturing analytics provides real-time contextual awareness. It gives decision-makers a competitive edge by digitizing the business, optimizing costs, improving quality, accelerating innovation, and redefining the customer experience. Manufacturing analytics is helping manufacturing companies increase the productivity and profitability of their operations by putting their massive amounts of data to work. Using machine learning models and data visualization tools, manufacturers are able to uncover insights in their data, optimize processes, and maximize performance.
Main business cases for manufacturing analytics
- Supply chain
- Demand forecasting
- Order management
- Inventory optimization
- Supplier performance
- Transportation analytics
- Early warning systems
- Product quality
- Real time quality monitoring
- Root cause
- Reliability
- Warranty
- Field Service & Support
- Inventory management
- Supplier performance
- Transportation analytics
- Creating an efficient factory
- Real time equipment & process monitoring
- Process capability
- Optimize maintenance
- OEE & factory productivity
The manufacturing analytics journey - from insights to action
But how do you accomplish these business goals? The manufacturing analytics journey is intended to turn the data that you gather from all of your manufacturing data into insights that can then be turned into actions that positively affect the business. As you can see in the chart below, the journey starts with identifying the business use cases. Most manufacturers have similar objectives that they are trying to reach including improving product quality and reliability, growing their revenue and creating an efficient factory. Let’s go left to right through the journey to learn all the steps to turn your manufacturing insights into actions.
After first identifying the business use cases, the next step in the journey is to assemble the data. Unfortunately, in manufacturing, there is so much data coming off of the factory floor, off of connected devices and sensors that data is often in silos. You have data for suppliers, processes, equipment, sales, and many other types of data as well. You need to wrangle that data, put it together, merge it, clean it, filter it if we need to and basically prepare it for analysis.
Once you do that, you can start to automate processes to look for signals such as defects, warranty claims, downtime or yield in the data. After we’ve done some initial exploration we may decide that there’s some standard ways we want to view things. We can create applications for real-time monitoring and dashboards that can be reused with new types of data.
Going beyond basic dashboards you can use advanced analytics applications to build models for further prediction based analyses. Some of your input data could be pressure, temperature, or product measurements. You can use models to check or predict production volumes, equipment failure and product quality.
Once we have a good predictive model, we want to be able to push out alerts. One example of alerts is onto mobile devices.
Goals of manufacturing analytics
The goal of manufacturing analytics is to go from a simple collection and display of data (descriptive) to being able to leverage that data in real time (predictive) for detection of issues with processes and equipment, reducing costs and maximizing efficiencies throughout the supply chain with less overhead and risk. Manufacturing analytics make those insights available to everyone from the CEO down to the shop floor worker.
Manufacturing analytics can help improve the quality of a company’s end product. It does this through several processes such as data-driven product optimization, managing defect density levels and analyzing customer feedback and purchasing trends. Data-driven product optimization can rely on IoT sensors and machine learning models to optimize production based on many factors. By analyzing product usage in detail, manufacturers can reduce or increase components that lead to higher usage rates. As a manufacturer, you must keep your defect density ratio low. With data collected from digital factories, manufacturers can now more specifically understand process states that lead to increased defect density. Customer analytics enable you to understand customers’ buying habits and lifestyle preferences. Armed with the information of future buying behaviors, manufacturers can more accurately produce and deliver what customers actually want.
Manufacturing analytics can also increase production yield and throughput. One of the main ways it does this is through anomaly detection. Anomaly detection can alert factory supervisors of defects in their products early on in production so they can resolve issues quickly and without affecting the output. Anomaly detection utilizes a combination of IoT sensors, historical data, and machine learning algorithms to detect unusual data which might be an indication of a developing problem.
Manufacturing analytics can also reduce risks and costs associated with downtime or equipment failures. This is accomplished by identifying bottlenecks or unprofitable production lines, and by anticipating failures and decreasing machine downtime to reduce costs with predictive maintenance of critical assets.
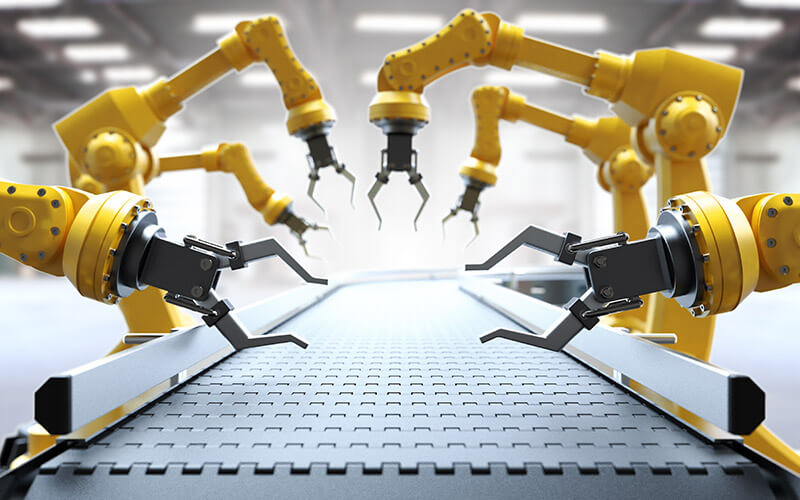